报告题目: What is next for Knowledge Graphs: Relevating the semantic Web vision
James Hendler(美国伦斯勒理工学院)
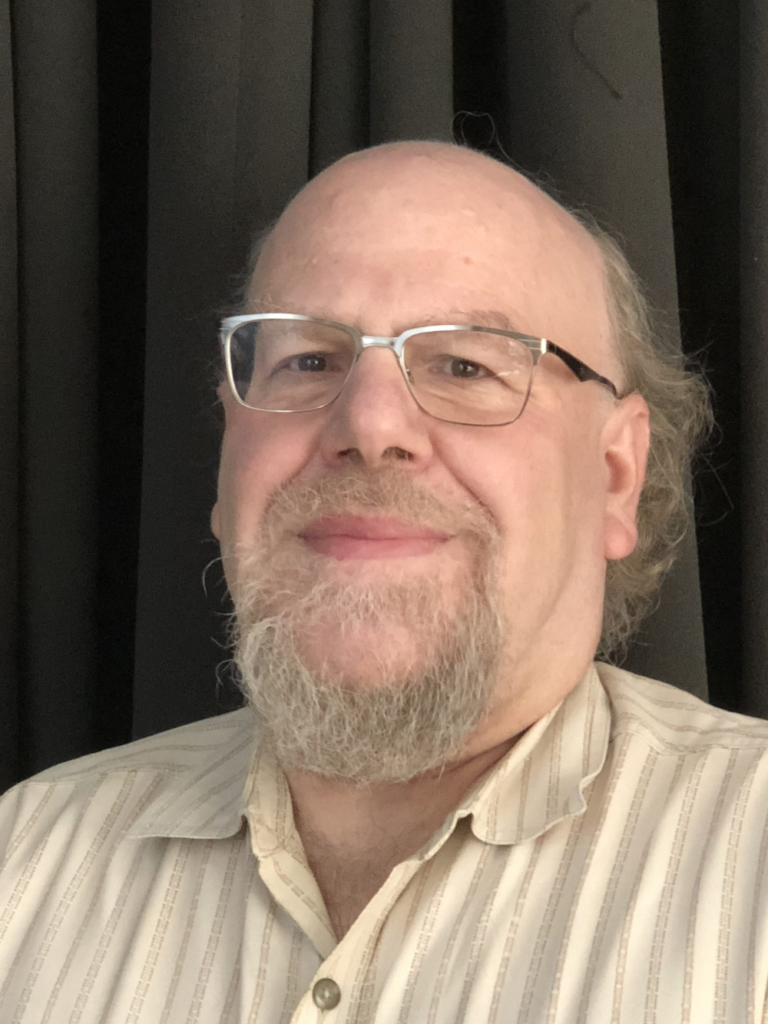
专家介绍:
James Hendler is the Director of the Future of Computing Institute and the Tetherless World Professor of Computer, Web and Cognitive Sciences at RPI. Hendler is a data scientist with specific interests in open government and scientific data, AI and machine learning, semantic data integration and the data policy in government. One of the originators of the Semantic Web, he has authored over 450 books, technical papers, and articles in the areas of Open Data, the Semantic Web, AI, and data policy and governance. Hendler is a Fellow of the AAAI, AAIA, AAAS, ACM, BCS, IEEE and the US National Academy of Public Administration.
报告摘要:
Knowledge Graphs have become a cornerstone in organizing and interpreting vast amounts of data, offering unprecedented insights and fostering innovation across various industries. As we stand on the cusp of the next evolution, this talk will explore the future directions and potential breakthroughs in Knowledge Graphs. We will delve into emerging trends such as the integration of machine learning with semantic technologies, enhancing interoperability, and scaling knowledge graphs for real-time applications. Additionally, we will discuss the role of advanced reasoning capabilities and the importance of contextual understanding in enriching the semantic vision. In this talk, we explore some of the original goals of the semantic web and knowledge graph technologies, some of their current uses, and some issues to consider for the future.
报告题目: 地学知识图谱
王新兵(上海交通大学)
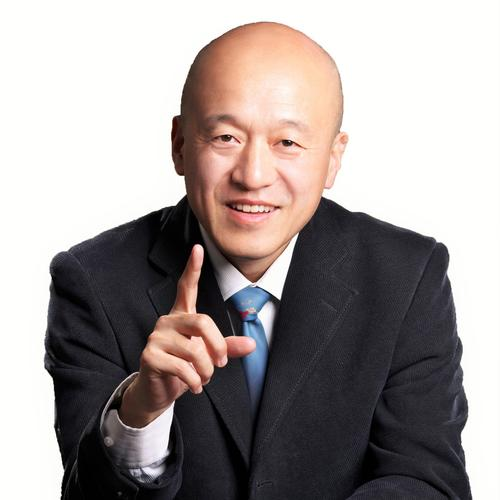
专家介绍:
王新兵,上海交通大学特聘教授,国家自然科学基金委杰出青年基金获得者,国家万人计划科技创新领军人才。现任ACM中国理事会主席(2021-),中国地质学会“数据驱动与地学发展专业委员会”副主任委员(2023-)。曾任上海交通大学电子信息与电气工程学院副院长,上海交通大学John Hopcroft计算机科学中心执行主任。担任多个CCF-A类国际期刊资深编委,包括IEEE Transactions on Information Theory, IEEE/ACM Transactions on Networking。发表领域A类期刊与会议论文100余篇,谷歌学术引用1万余次,连续六年入选爱思唯尔(Elsevier)中国高被引学者。2012年获中国计算机学会青年科学家奖,2018年获高等教育国家级教学成果奖二等奖(排2)。累计培养57位学生(其中优博3位)在高校任教职,包括C9高校17位、985高校26位、8位担任院长/系主任职务。
报告摘要:
地球科学与信息科学的深度交叉融合,为人工智能助力的地学研究、深时空数据驱动的知识图谱构建带来了机遇和挑战。一方面,信息科学的人工智能、大数据、网络信息等领域的飞速发展,为地学研究的科学发现提供了新手段;另一方面,地学大数据的深时空(深时、深空、深地、深海)特性,拓展了知识图谱演化必需的数据多样性。地学知识图谱已成为地球科学与信息科学的国际前沿交叉研究热点。围绕深时空数据与知识图谱之间的表征同构映射、互促作用机理等科学问题,开展地学数据的结构化表示重建、知识图谱的深时空进化生长、图谱驱动的知识计算及发现等基础研究,能有效助力大数据时代的地学科学发现。
报告题目: 星火大模型最新进展及典型行业应用
王士进(讯飞研究院)
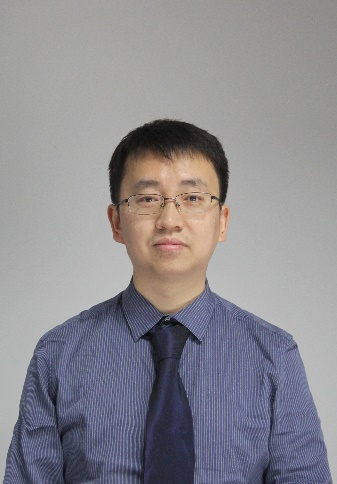
专家介绍:
王士进博士,正高级工程师,现任讯飞研究院执行院长、认知智能全国重点实验室副主任。他主要从事信号处理、大模型、行业智能等研究,获国家科技进步一等奖、省科学技术奖一等奖、吴文俊人工智能科技进步奖一等奖、中国青年五四奖章集体、中国科协求是杰出青年成果转化奖,带领团队获得 SQuAD、GLUE、VCR等十几项国际比赛冠军。他主导发布了行业领先的星火认知大模型,并持续赋能多个行业应用。
报告摘要:
本报告首先提出当前以认知大模型为代表的通用人工智能技术引发全球广泛关注,然后还分析了从认知大模型到多模态大模型的技术特性、发展趋势及应用价值。其次,报告汇报了科大讯飞研发星火大模型的成果和研发经历,最后重点介绍了大模型服务典型行业的探索经验。
报告题目: Semantic Aware Machine Learning and Explanations for Knowledge Graphs
Claudia d’Amato(意大利巴里大学)
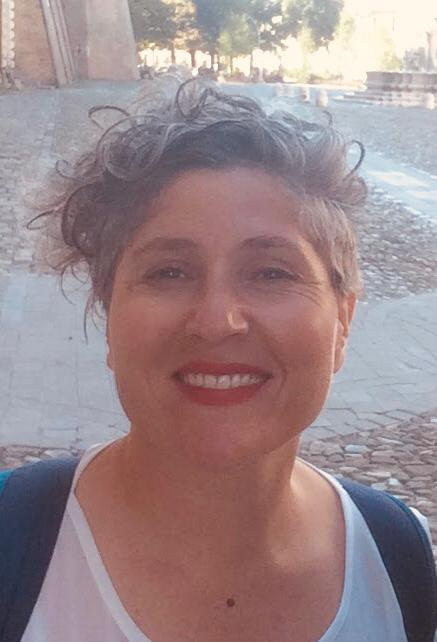
专家介绍:
Claudia d’Amato is associate professor at the University of Bari – Computer Science Department and she got the Italian Habilitation for the functions of Full Professor for the Scientific Sector “09/H1 – Information Processing Systems” (currently GDS 09/IINF-05 – SISTEMI DI ELABORAZIONE DELLE INFORMAZIONI) on April 14th, 2021 and for the Scientific Sector “01/B1 – Informatics” (currently GDS 01/INFO-01 – INFORMATICA) on April 29th, 2021. She obtained her PhD in 2007 from the University of Bari, Italy, defending the thesis titled “Similarity Based Learning Methods for the Semantic Web. She pioneered the research on Machine Learning methods for ontology mining and Knowledge Graphs that still represents her main research interest jointly with the development of neural-symbolic and explainable solutions to be applied to Knowledge Graphs. Claudia d’Amato has been also invited researcher at several universities and international research institutes such as: the University of Koblenz-Landau in 2006, 2007, 2008, 2013 working with Prof. Stefen Staab, the University of Oxford in 2012 working with Prof. Thomas Lukasiewicz, INRIA –Sophia-Antipolis in 2015 working with Dr. Fabien Gandon and Prof. Andrea Tettamanzi, the University of Poznan in 2011 and 2013 working with Dr. Agnieszka Lawrynowicz, FBK in 2012 working with Dr. Luciano Serafini. She is member of the editorial board of the Transactions on Graph Data and Knowledge (TGDK) journal, the Neurosymbolic Artificial Intelligence Journal, the Semantic Web Journal and the Journal of Web Semantics. She served as General Chair for ISWC 2022, Program Chair for ISWC 2017, ESWC 2014, Vice-Chair for ISWC 2009, Journal Track chair for TheWebConf 2018 (previously WWW), Tutorial Chair for ECAI 2020, Machine Learning Track Chair for ESWC’12- ’13-’16-’17 and PhD Symposium chair at ESWC’15-’21 and at ISWC’23. She served/is serving as a program committee member of a number of international conferences in the area of Artificial Intelligence, Machine Learning and Semantic Web such as AAAI, IJCAI, ECAI, ECML, ISWC, TheWebConf, ESWC.
报告摘要:
Knowledge Graphs (KGs) are receiving increasing attention both from academia and industry, as they represent a source of structured knowledge of unprecedented dimension to be exploited in a multitude of application domains as well as research fields. Nevertheless, despite their large usage, it is well known that KGs suffer of incompleteness and noise since they often come as a result of a complex building process. As such non-negligible research efforts are currently devoted to improve the coverage and quality of existing KGs. Particularly, for the purpose numeric based Machine Learning (ML) solutions are generally adopted, given their proved ability to scale on very large KGs. Numeric-based approaches mostly focus on the graph structure and they generally consist of series of numbers without any obvious human interpretation, thus possibly affecting the interpretability, the explainability and sometimes the trustworthiness of the results. Nevertheless, KGs may also rely on expressive representation languages, e.g. RDFS and OWL, that are also endowed with deductive reasoning capabilities. However, both expressiveness and reasoning are most of the time disregarded by the majority of the numeric methods that have been developed, thus somehow loosing knowledge that is already available. In this talk, the role and the value added that the semantics may have for ML solutions as well as for providing explanations to tasks such as link prediction will be argued. Hence, the research directions on empowering ML and explanation solutions by injecting background knowledge will be presented jointly with the analysis of the most urgent issues that need to be solved.