DATE | TIME | TUTORIAL | SPEAKER | CHAIR |
---|---|---|---|---|
August 24th morning | 8:30–10:00 | Research and Prospect of Multimodal Knowledge Graph | Zhixu Li | Ming Liu |
10:30-12:00 | Research on Multimodal Semantic Structuration | Tong Xu | ||
August 24th afternoon | 14:00-15:30 | Efficient Knowledge Graph Construction and Reasoning | Ningyu Zhang, Wen Zhang | |
16:00-17:30 | Trustworthy Natural Language Understanding under the Background of Big Models | Xiao Ding | ||
August 25th morning | 8:30-12:00 | Conversational AI: Progress and Trends | Lu Chen | Qili Zhu |
August 25th afternoon | 14:00-17:30 | Review and Prospect of Unsupervised Machine Translation | Rui Wang |
T1
Research and Prospect of Multimodal Knowledge Graph
Abstract:Multimodal Intelligence is one of the mainstream trends in the current development of Artificial Intelligence. The core of Multimodal Intelligence is Cross-modal Knowledge Engineering and Cognitive Intelligence, which mainly involves researches on multimodal knowledge acquisition, representation and reasoning. This tutorial will focus on the introduction of the current research status of Multimodal Knowledge Graph and Cross-Modal Cognitive Intelligence, by sharing and prospecting the research hotspots, key challenges and future directions.
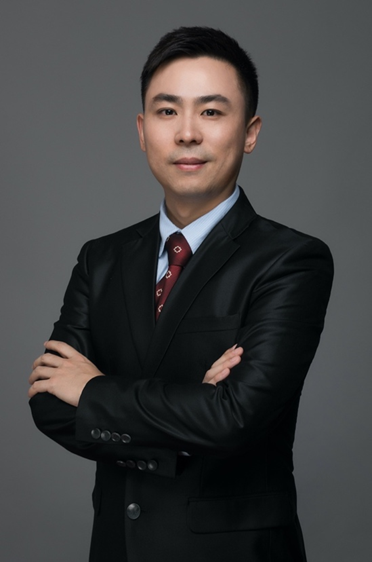
Bio:Zhixu Li is a researcher and doctoral supervisor at School of Computer Science and Technology, Fudan University, and an associate director of the State Key Laboratory of Cognitive Intelligence (Suzhou). He got his Ph.D. in Computer Science from the University of Queensland, Australia. His main research interests include knowledge graph, cognitive intelligence, big data analysis and mining. He has published more than 150 papers in mainstream journals and international conferences such as TKDE, ICDE, IJCAI, AAAI, ACL, CIKM, WSDM, ISWC, WWWJ, DASFAA etc., and presided more than 10 scientific research projects and school-enterprise cooperation projects.
T2
Research on Multimodal Semantic Structuration
Abstract:Multimodal information, with its more diverse information presentation, has become increasingly important in the application of artificial intelligence. However, it also raised greater challenges on semantic understanding and knowledge extraction tasks due to the non-structural property. Recently, with the accumulation of corpus and technical tools, semantic structuration of multimodal information could be available via the deep fusion of graph neural network technology and multimodal information. In this talk, we will first discuss the challenges and process for the semantic structuration of multimodal information, and then introduce the corresponding intelligent applications, as well as the future directions.
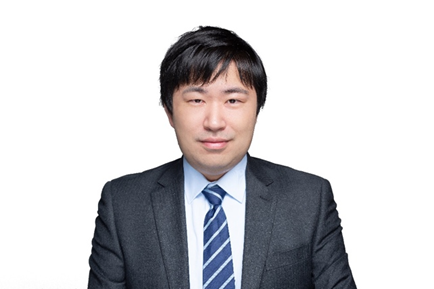
Bio: Tong Xu is currently working as an Associate Professor of University of Science and Technology of China. Also, he serves as an executive member of the Youth Working Committee and Committee of Affective Computing for CIPS, as well as a member of the Committee of Social Media Processing, CIPS. He has published more than 50 technical papers on CCF rank-A journals or conferences, including IEEE TKDE、ACM TOIS、KDD、WWW、ACM MM, etc. He has won two best papers award (or nominations) for international conferences, and 7 awards for top-tier challenges including CVPR, ACM MM, etc. He won the ACF Award for Outstanding Young Scientist in 2022.
T3
Efficient Knowledge Graph Construction and Reasoning
Abstract:Knowledge graphs (KGs) are large-scale semantic networks that use a graph model to describe knowledge and model the relationship between all things in the world. KG construction aims to extract entities, relations, attributes and events from massive structured and unstructured data, store knowledge in KGs, and conduct knowledge reasoning for applications. This tutorial will focus on introducing the cutting-edge research of efficient knowledge graph construction and reasoning, sharing the studies related to this direction, including meta-learning, pre-training, prompt learning, graph neural network, rule mining, etc., and look forward to the future research directions. This tutorial will also introduce the knowledge graph extraction tool (DeepKE) and knowledge graph reasoning tool (NeuralKG).

Bio: Ningyu Zhang, Ph.D., associate professor at Zhejiang University, his main research interests are NLP, knowledge graphs, etc. He has published papers in top international academic conferences and journals such as ICLR、ACL、EMNLP、NAACL、KDD、WWW、AAAI、TASLP, with more than 1,000 Google Scholar citations. He has served as a program committee member for many top international academic conferences, including ACL、NeurIPS、ICLR、ICML、KDD、AAAI. He has won the second prize of the Zhejiang Science and Technology Progress Award, CCKS2021 best paper award, and IJCKG2021 best paper nominations. He has served as a member of the Youth Working Committee of the CIPS and the Language and Knowledge Computing Professional Committee of the CIPS.
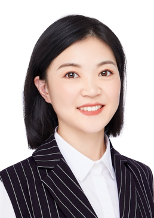
Bio: Wen Zhang is a researcher at the School of Software Technology at Zhejiang University. Her research interests include knowledge graph, big data intelligence, and graph computing. She has published over 30 research papers about representation learning, rule learning, and pre-training at international academic conferences, including WWW, ICDE, SIGIR, KDD, and IJCAI.
T4
Trustworthy Natural Language Understanding under the Background of Big Models
Abstract:Currently, data-driven large-scale pre-trained natural language understanding models represented by BERT and GPT have achieved impressive performances on multiple natural language understanding tasks, becoming a new paradigm for natural language understanding. However, the natural language processing results given by the current big models are still unreliable, which is mainly reflected in three aspects: poor model stability, weak interpretability, and insufficient generalization ability. This tutorial addresses the problem of how to do trustworthy natural language understanding on the background of big models. First, in order to solve the problem of poor stability of big models, this tutorial introduces a stable natural language understanding method guided by a causal mechanism. Secondly, in order to solve the problem of weak interpretability of large models, this tutorial introduces an interpretable natural language understanding method based on neural-symbolic methods. Finally, in order to solve the problem of insufficient generalization of large models, this tutorial introduces a generalizable big model training architecture for low resources.
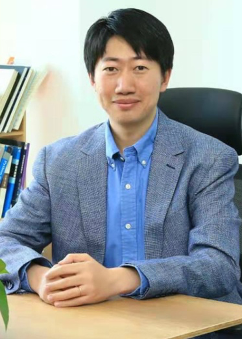
Bio: Xiao Ding, associate researcher and doctoral supervisor of Harbin Institute of Technology. The main research directions are artificial intelligence, natural language processing, cognitive reasoning and eventic graph. He has published more than 40 papers in famous international journals and conferences in the field of artificial intelligence such as ACL, AAAI, IJCAI, EMNLP, TASLP, etc. Undertook a number of projects above the provincial and ministerial level such as the National Natural Science Foundation of a research project. He won the second prize of Heilongjiang Science and Technology Progress Award, the first place in the task of “Detecting Counterfactual Statements” in the SemEval 2020 International Semantic Evaluation, and was selected as the 2022 AI 2000 Most Influential Scholar in Global Artificial Intelligence (AMiner selection). He is the deputy director of the Heilongjiang Provincial Key Laboratory of Chinese Information Processing, a member of the Standing Committee of the Social Media Processing Special Committee of the Chinese Information Society, a member of the Language and Knowledge Computing Special Committee, and a member of the Youth Working Committee of the Chinese Information Society of China.
T5
Conversational AI: Progress and Trends
Abstract:In recent years, conversational AI technology has been widely used in intelligent customer service, intelligent IoT things, intelligent voice assistants, and other fields. With the rapid development of pre-trained language models, the capabilities of natural language understanding, response generation, and knowledge infusion of dialogue systems have been greatly improved. In this tutorial, we will introduce the latest progress in dialogue understanding and generation, and knowledge infusion, and will focus on the pre-trained dialogue models for different dialogue tasks. Finally, we will discuss the current challenges and future trends of dialogue systems.
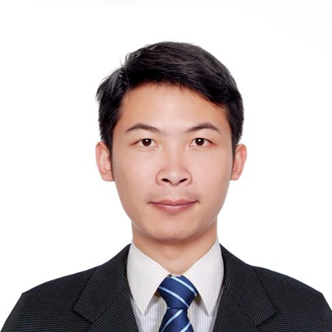
Bio: Dr. Lu Chen is an assistant research professor at the department of computer science and engineering, Shanghai Jiao Tong University (SJTU). His research interests include dialogue systems, question answering, and natural language processing. He has published more than 30 journal articles (e.g. IEEE/ACM Transactions) and peer-reviewed conference papers (e.g. ACL, EMNLP, NAACL, AAAI), one of them was selected as COLING2018 Area Chair Favorites. He also received the 2020 SJTU Outstanding Doctoral Dissertation Award and was nominated for the 2021 CCF Outstanding Doctoral Dissertation Award.
T6
Review and Prospect of Unsupervised Machine Translation
Abstract: Machine translation (MT) is a classic topic in NLP and AI. From 2010s when abundant compu-tational resources became available, neural machine translation (NMT) reached state-of-the-art performance. Recently, unsupervised NMT (UNMT), which only relies on monolingual corpo-ra, has achieved impressive results. Meanwhile, there are still several challenges for UNMT. This talk first introduces the background and the latest progress of UNMT. We then examine a number of challenges to UNMT and give empirical results on how well the technology current-ly holds up.
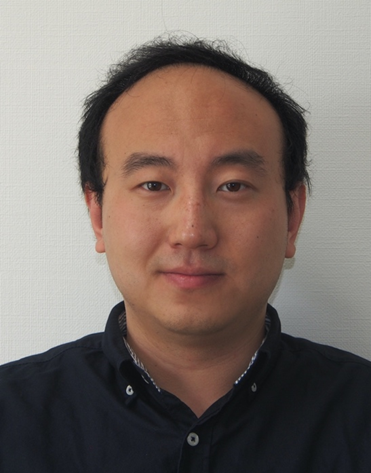
Bio: Dr. Rui Wang is a computational linguist working as an associate professor at Shanghai Jiao Tong University since 2021. Before that, he was a researcher (tenured in 2020) at Japan National Institute of Information and Communications Technology (NICT) from 2016 to 2020. His research interest is NLP, especially machine translation (MT). He has published more than 40 papers in top-tier NLP/ML/AI conferences and journals, such as ACL, EMNLP, ICLR, AAAI, IJCAI, TPAMI, TASLP, etc. He served as the area chairs of NeuRIPS-2022, ICLR-2021/2022, EMNLP-2022, NAACL-2021, etc. He gave cutting-edge tutorials at EACL-2021 and EMNLP-2021. He has also won several first places in top-tier MT/NLP shared tasks, such as WMT-2018, WMT-2019, WMT-2020, CoNLL-2019, etc. Its related technology is widely used in VoiceTra, the official machine translation software of the Tokyo Olympics.