Open Knowledge Graph Canonicalization
Abstract: Open information extraction technology extracts factual triples in the form of <noun phrase, relational phrase, noun phrase> from the text to form open knowledge graphs, which are large scale, wide coverage and fast updating. However, noun phrases and relational phrases in open knowledge graphs are not canonicalized and lack unique identifiers, resulting in a large number of redundant and scattered facts stored in them, which hinders their use in downstream applications. The task of open knowledge graph canonicalization is to cluster noun phrases and relational phrases with the same semantics into the same cluster and assign unique identifiers to them. This report will present our recent work on the open knowledge graph canonicalization task.
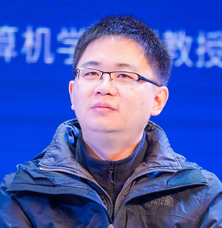
Bio: Wei Shen is an associate professor at Nankai University. His research interests are data mining and knowledge graphs. He has published more than 10 papers as the first author or corresponding author in top international conference journals (ACM SIGMOD, ACM SIGKDD, WWW, IEEE ICDE, IJCAI, IEEE TKDE) in the fields of data mining, database, and artificial intelligence. He has won the China Association for Science and Technology Young Talent Support Project, the ACM China Rising Star Award Nomination Award, the CCF-Intel Young Scholars Promotion Program, the China Association for Artificial Intelligence Outstanding Doctoral Dissertation Award, and the Google Global Doctoral Fellowship.
Event Knowledge Graph Construction
Abstract: Recently, event-centric knowledge graphs have received a lot of attention, they can be applied to various tasks acquiring commonsense reasoning, such as reading comprehension, event prediction, and story completion. Events have stronger representation ability than entities in traditional knowledge graphs. An event can describe either a fact or process that is happening or has happened, or a momentary state at a certain moment. However, extracting events and relations from text faces new challenges. First, the knowledge of events is more complex, including trigger words, event elements, and element roles, etc. Second, different events and their elements are often scattered through the documents, which requires long texts understanding and cross sentences reasoning. Even state-of-the-art large-scale pretrained models cannot generate satisfactory results. This report will share some new technologies of event and relation extraction at the document level, which can handle the above challenges. This report will also introduce the construction process of the event knowledge graph.
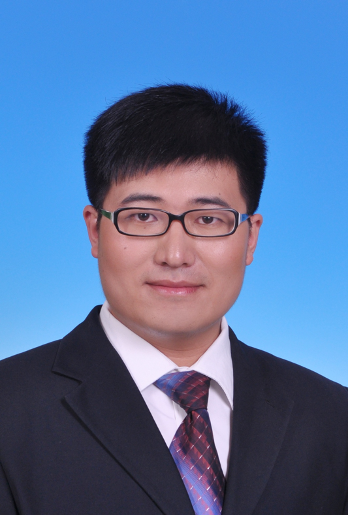
Bio: Yixin Cao is an assistant professor at Singapore Management University. His research interests include knowledge graph and natural language processing. He obtained his Ph.D. from Tsinghua University in 2018. After graduation, he did post-doctoral research at the National University of Singapore, and then worked as a research assistant professor at Nanyang Technological University. He has published more than 30 papers in international authoritative conferences and journals, his papers have been cited more than 2,600 times according to Google scholar. He has served as PC member in several top conferences, and hosted and participated in a number of projects funded by Singapore government.
Construction and Application of Educational Knowledge Graph
Abstract: Educational knowledge graphs are constructed for the field of education, which aim to represent different elements involved in the teaching process and various cognitive relationships between these elements. Educational knowledge graphs are the core of intelligent education systems, which enable the aggregation of heterogeneous learning resources, the organization of massive learning resources, knowledge association, group collaborative learning, personalized learning, and adaptive learning. This report will discuss how to build an education-oriented knowledge graph to meet the practical needs of education and teaching, and the typical smart education applications based on educational knowledge graphs.
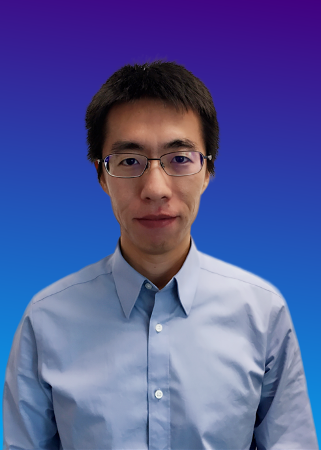
Bio: Penghe Chen is a lecturer of the Faculty of Education, Beijing Normal University, he is also the Deputy Director of the Artificial Intelligence Laboratory of the Future Education Advanced Innovation Center of Beijing Normal University. He graduated from the National University of Singapore with a Ph.D., and mainly conducts research on educational knowledge graphs, artificial intelligence education applications, and intelligent education assistants. He has published a number of high-level research papers in the field of artificial intelligence and educational technology, and hosted a number of research projects including the NSFC projects.