Title 1: Knowledge Graph Construction and Reasoning: Recent Progress and Opportunities
Abstract:Knowledge graph (KG) construction and reasoning are research fields of great significance, aiming to construct and reason over rich KGs for better knowledge understanding and processing. Recent years has witnessed significant progress in KG construction and reasoning. This talk will focus on several important trends, including end-to-end KG construction and reasoning, modularization of tools for complex KG construction and reasoning, diverse types of reasoning, multimodal data integration, and KG construction and reasoning that combines implicit knowledge from LLMs and multi-agent collaboration. Furthermore, this report will also provide a brief overview of the latest developments in industrial applications of KG construction and reasoning.
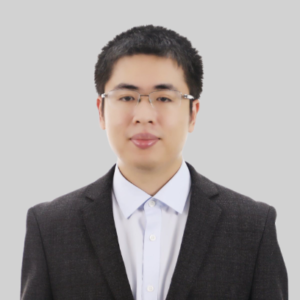
Bio:Dr. Ningyu Zhang is an associate professor at Zhejiang University. His research interests include knowledge graph and natural language processing. He has published multiple top conference papers and journal articles in the field of knowledge graph and natural language processing, including ACL, EMNLP, NAACL, NeurIPS, ICLR, and more. His work has been cited over two thousand times on Google Scholar, and four of his papers have been selected as Paper Digest Most Influential Paper. He has received the second prize of Zhejiang Province Science and Technology Progress Award and has been awarded the Best Paper or Best Paper Nomination at the IJCKG and CCKS. He has served as the program chair for ACL and EMNLP, an action editor for ARR, a senior program committee member for IJCAI, an associate editor for ACM Transactions on Asian and Low-Resource Language Information Processing, and a program committee member for conferences such as NeurIPS, ICLR, and ICML. He is also a member of the Language and Knowledge Computing Committee and the Youth Working Committee of the Chinese Information Processing Society of China.
Title 2: Knowledge-Enhanced Large-Scale Language Models
Abstract:With the development of large-scale language models and generative artificial intelligence technology, large conversational models such as ChatGPT have attracted widespread attention in academia and industry. They have been successfully applied in various scenarios such as information retrieval, code generation, intelligent customer service, and text editing. While the immense size of these models and their massive unsupervised pretraining corpora enable them to store vast amounts of the general domain and specialized knowledge, as well as exhibit excellent text generation and language understanding capabilities, they still face challenges related to fabricating facts and producing misleading statements. This talk will focus on the integration of external knowledge bases and the mining and refinement of internal knowledge within large language models, which significantly improve the issues of fabricating facts and generating misleading statements. Furthermore, by integrating with knowledge bases, the complex reasoning and continual learning abilities of large models can be further enhanced.
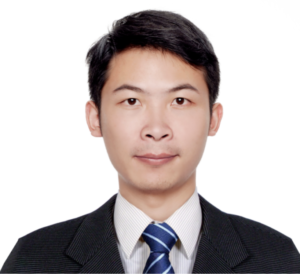
Bio:Dr. Lu Chen is an Assistant Research Professor in the Department of Computer Science and Engineering at Shanghai Jiao Tong University. His research interests include intelligent dialogue and question-answering systems, natural language processing, etc. He has published over 40 papers in major international conferences and journals in the field of natural language processing, such as TACL, ACL, EMNLP, and NAACL. He has received COLING2018 Area Chair Favorites, NCMMSC2022 Best Paper Award, and a nomination for 2021 CCF Outstanding Ph.D. Thesis Award. He has participated in some international challenges in the field of intelligent human-computer dialogue and question answering, such as DSTC, Spider, CSpider, and CBLUE2.0, winning championships or achieving first place. Some of his research outcomes have been widely applied in the industry and He received the 23rd China Patent Award.