报告1
自然语言处理面临的重要挑战与机遇
孙茂松(清华大学)
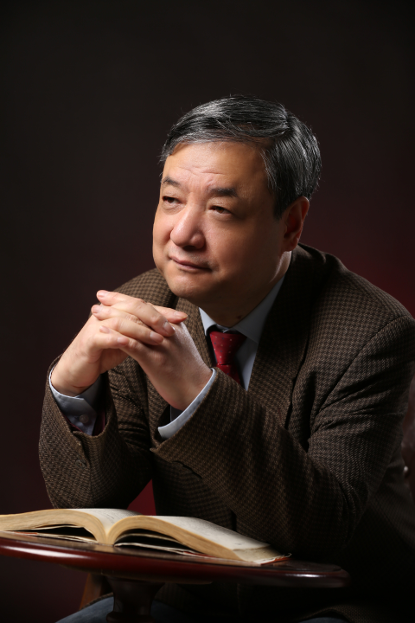
讲者介绍:
孙茂松,清华大学计算机科学与技术系长聘教授(2007-2010年任该系系主任),清华大学人工智能研究院常务副院长,欧洲科学院外籍院士、中国人工智能学会会士、中国中文信息学会会士。主要研究领域为自然语言处理,人工智能,社会、人文及艺术计算。国家重点基础研究发展计划(973计划)项目首席科学家,国家社会科学基金重大项目首席专家。在重要国际刊物、国际会议、国内核心刊物上发表论文200余篇,Google Scholar论文引用2.5万余次。现任学术兼职包括教育部教学信息化与教学方法创新指导委员会副主任委员,国家语言文字工作委员会第三届科研规划领导小组顾问,《中文信息学报》主编,《数字人文》共同主编,中央音乐学院兼职教授和博士生导师,新加坡国立大学访问教授等。2016年获“全国优秀科技工作者”。
报告摘要:
当前自然语言处理研究正面临着来自两个方面的重要挑战:一方面,近年来以BERT和GPT3为代表和发端的大规模预训练语言模型,“出乎意料”地成为世界范围内整个人工智能领域技术竞争的战略焦点和热点,引领了一个时期的潮流(如各类预训练语言模型乃至预训练多模态模型如雨后春笋般层出不穷)。基于深度学习的自然语言处理正沿着“极大数据、极大模型、极大算力”的轨道“无所不用其极”地一路奋进,产生了若干令人感到惊异但同时也多少有些令人迷惑不解的现象。然而,这条路貌似已走到极致,下一步该怎么走呢?另一方面,人们在日益认识到大模型局限性的同时,也愈加认识到以知识图谱为典型代表的大规模形式化知识系统在智能计算中的关键作用。但知识图谱建设仍然存在着结构性短板,对智能计算相关研究及应用形成了严重制约,亟待我们克服。那么,在此“挑战”形势下,自然语言处理领域有哪些新的机遇呢?本报告将围绕上述问题展开初步的讨论。
报告2
Data-Centric University 4.0
Hong-Gee Kim (Seoul National University)
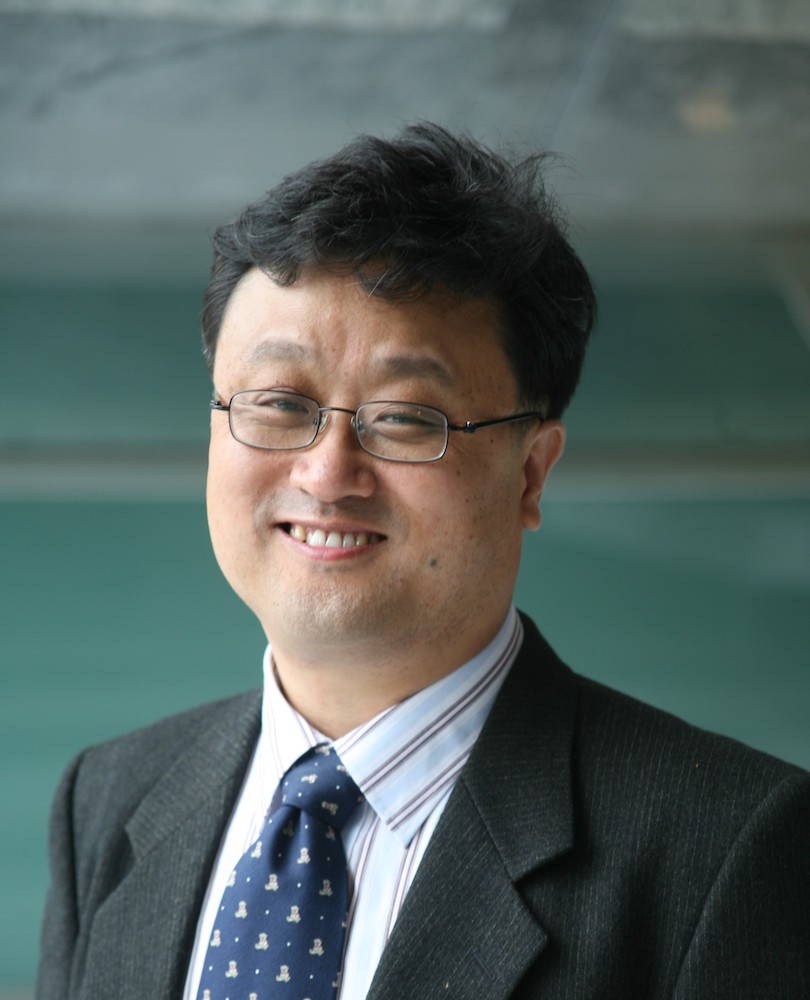
Bio:
Prof. Hong-gee Kim is the CIO of Seoul National University. He is also the Chair of KREN (the Korean Education Network) representing 400 higher education institutes in Korea. He has a professorship at Seoul National University as the head of Dental Management & Informatics department. He also has joint appointments at Computer Engineering Dept, Cognitive Science Dept, and Archival Studies Dept of Seoul National University. Overseas, he was an adjunct professor in Information and Engineering School, the National University of Ireland, Galway. During his 2 sabbatical leaves, he visited Harvard University Medical School and Helsinki University Medical School respectively.
Hong-Gee Kim has authored over 300 research papers and 7 books that cover diverse topics in computer engineering, clinical medicine & dentistry, bioinformatics, cognitive science, law, and industrial engineering. His current research interests include data-centric biology & medicine, semantic technologies for large scale biomedical data integration, deep data analysis with various machine learning technologies for cancer and epigenetic informatics. One of his main research topics is to develop AI-based software tools for drug discovery in the context of precision medicine. For this his lab makes efforts to integrate diverse biological data with large-scale disease networks using knowledge graphs. In addition to research interests, Hong-Gee Kim is deeply involved in digital transformation in Korean higher education systems. Recently, he organized and launched a consortium, named Big Data COSS (Convergence Open Sharing System) with 7 universities to build an innovative open university system to facilitate data-centric disciplines in all subjects.
Abstract:
With COVID-19 spreading around the world, many countries have used and developed various medium, digital solutions and information infrastructure for distance education. The dramatic change caused by the pandemic speeded up adopting new technologies in higher education and revolutionizing university systems. The University 4.0 paradigm, which is inspired by Industry 4.0, comes up with more effective responses to the demand for improvement, optimization, and personalization of large-scale data-centric and technology-supported education. In this talk, I will explore how the data-centric approach to University 4.0 changes the structure and processes of university systems from several different aspects. Firstly, we can reconceptualize University as a Platform and Education as a Service. A data-centric learning commons platform and MOOCs will be more popularly used for higher education. Secondly, University 4.0 means the shift towards more student-centric universities that can provide competency-based educational services. Precision education that can be adapted to learner’s capability would require various computational tools to effectively manage and analyze a large amount of learner’s information. Thirdly, University 4.0 facilitates globalized open innovation for collaborative research. Open data platforms across many fields and AI tools are changing the landscape of scientific research where there is no boundary between universities and between academia and industry.
One of the most important features of the data-centric University 4.0 is that the educational achievement criteria shift from how much a learner has spent time in the classroom (Time-based Education) to how much a learner has actually acquired the targeting ability (Competency Based Education, CBE). For efficient CBE, it is required to systematically manage a large amount of learner’s information related to classroom activities, background knowledge, future goals, etc. One of the most notable data frameworks for CBE is a knowledge graph (or linked data) model that can manage the learner’s competency by linking information such as student’s capabilities, educational resources, learning targets, and referential meta-information. In this talk I will present some recent technologies of knowledge graphs that propose to link various datasets regarding structured competency data, classroom activities, course syllabus, and educational resources within a university or cross multi universities. I will also briefly introduce a recent linked data model to store and manage student’s personal information without worrying about the invasion of privacy.
报告3
Inference in Open-Domain Question-Answering
Mark Steedman (The University of Edinburgh)
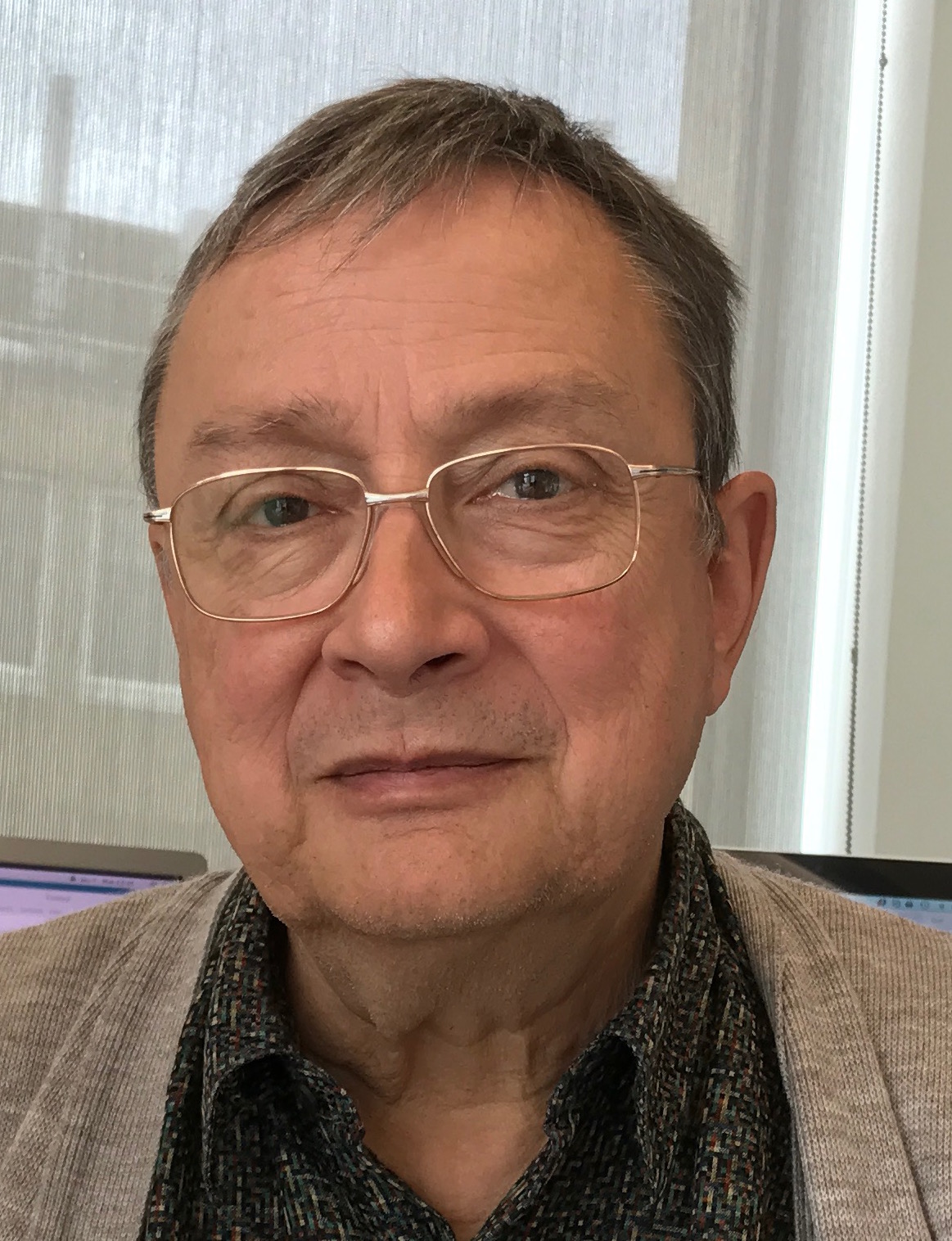
Bio:
Mark Steedman is Professor of Cognitive Science in the School of Informatics at the University of Edinburgh, to which he moved in 1998 from the University of Pennsylvania, where he taught for many years as Professor in the Department of Computer and Information Science. He is a Fellow of the British Academy, the Royal Society of Edinburgh, the American Association for Artificial Intelligence (AAAI), the Association for Computational Linguistics (ACL), and the Cognitive Science Society (CSS), and a Member of the European Academy. In 2018, he was the recipient of the ACL Lifetime Achievement Award.
His research covers a wide range of problems in computational linguistics, natural language processing, artificial intelligence, and cognitive science, including syntactic and semantic theory, and parsing and interpretation of natural language text and discourse, including spoken intonation, by humans and by machine. Much of his current research uses Combinatory Categorial Grammar (CCG) as a formalism to address problems in wide-coverage parsing for robust semantic interpretation and natural language inference, and the problem of inducing and generalizing semantic parsers, both from data and in child language acquisition. Some of his research concerns the analysis of music using related grammars and statistical parsing models.
Abstract:
Open-domain question-answering from text corpora like Wikipedia and the Common Crawl generally requires inference. Perhaps the question is “Who owns Twitter?”, but the text only talks about people buying (or not buying) that company. To answer the question, we need a structure of “meaning postulates” that includes one that says buying entails ownership. Such structures are commonly (though inaccurately) referred to as “entailment graphs (EG).” They are inherently directional: the fact Twitter Inc, owns Twitter does not answer the question “Who bought Twitter?”.
Two approaches are currently being pursued. One approach is to hope that large large models (LM) can be fine-tuned for use as “latent” entailment graphs. I’ll argue following work by Javad Hosseini, Sabine Weber, and Tianyi Li that we see no evidence so far that LMs can learn directional entailment (as opposed to bidirectional similarity).
An alternative approach uses machine-reading with parsers over multiply-sourced text to extract a Knowledge Graph (KG) of relational triples representing events or relations that hold between typed entities, including buying and owning relations. We then build a (different) Entailment Graph (EG) on the basis of distributional inclusion between the triples. Such entailment graphs gain in precision, because they are inherently directional. They are scalable, and can be built for any language for which a reliable parser and named-entity linker is available. However, they are inherently sparse, because of the Zipfian Distribution of Everything in NLP.
I’ll discuss some recent work by Nick McKenna and the group investigating the theory of smoothing EGs using LMs, and use of WordNet/BabelNet to investigate further distributional asymmetries.